RAG, or Retrieval-Augmented Generation, is an effective technique for making AI systems smarter, more accurate, more up to date. It combines two major strengths: information retrieval and text creation. This novel approach assures that AI systems provide better and more dependable responses.
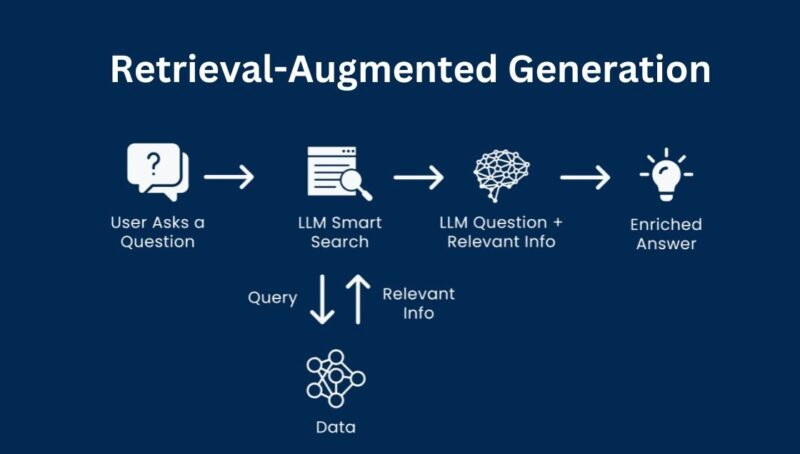
How Does RAG Work?
- Step 1: Search for Knowledge
When you ask a question, RAG first searches a knowledge base (like a library or database) to find relevant information. - Step 2: Combine and Create
It then uses this information, along with what it already knows, to generate a clear and accurate response. - Step 3: Give You the Answer
The final result is an answer that feels natural but is also grounded in reliable data.
RAG: The Future of Smarter AI Systems
RAG, or Retrieval-Augmented Generation, is a powerful way to make AI systems smarter, more accurate, and up-to-date. It combines two key strengths: finding information (retrieval) and creating meaningful text (generation). This unique approach ensures AI systems deliver better and more reliable answers.
How Does RAG Work?
- Step 1: Search for Knowledge
When you ask a question, RAG first searches a knowledge base (like a library or database) to find relevant information. - Step 2: Combine and Create
It then uses this information, along with what it already knows, to generate a clear and accurate response. - Step 3: Give You the Answer
The final result is an answer that feels natural but is also grounded in reliable data.
Why Use RAG Instead of Fine-Tuning?
RAG has many benefits compared to fine-tuning, which is another common way to improve AI models.
1 . Always Up-to-Date
RAG: You may update the knowledge base without retraining the model.
Fine-tuning: Requires new training each time you add or update knowledge.
- Handle new topics. Easily
RAG: Simply add the new topic to the Knowledge Base.
Fine-tuning necessitates the collection of new data as well as the retraining of the entire model. - Saves time and money.
RAG: There is no need for extensive training because it focuses on collecting useful information.
Fine-tuning: Retraining requires a large amount of time, processing power, and money. - Improved accuracy for specific questions
RAG: Retrieves the exact information required for the inquiry.
Fine-tuning: If the training data does not cover the issue, the response may be inadequate.
Real-Life Uses for RAG
- Customer Support: Provide quick and accurate answers by pulling from updated FAQs.
- Healthcare: Use the latest medical guidelines to help patients.
- Education: Deliver updated learning materials to students.
- Finance: Fetch real-time data to offer better investment advice.
Why RAG Is a Big Deal
RAG is changing how we think about AI. Instead of relying only on what the AI has been trained on, it lets the system bring in new information whenever needed. This makes AI more reliable, flexible, and ready for the future.
Conclusion
If you want AI systems that are smarter, faster, and more cost-efficient, RAG is the way to go. It’s especially useful in areas where things change often, like healthcare, education, and finance. With RAG, we’re moving toward an AI that doesn’t just understand the past—it’s ready for the present and the future.
Reference
https://research.ibm.com/blog/retrieval-augmented-generation-RAG
https://cloud.google.com/use-cases/retrieval-augmented-generation